Blog Responsible and Ethical Use of Data, Machine Learning, and AI
Responsible and Ethical Use of Data, Machine Learning, and AI
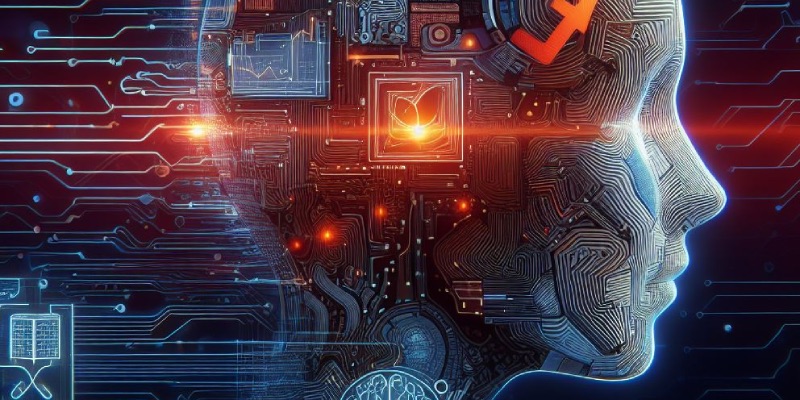
Data, machine learning, and AI have changed the world in many ways. And they’re just getting started. No one knows the limits of their transformative potential, but the massive risks they create already confound policymakers, expose businesses to legal liabilities, and undermine the ties that bind communities. Whether advanced AI constitutes an existential threat or holds the key to a technological utopia continues to be debated. But everyone agrees: data’s responsible and ethical use in all its forms — from a user’s private information to complex machine learning systems — is the only secure way forward.
Nearly every aspect of modern society has benefited tremendously from AI and machine learning. But serious flaws occasionally bubble up to the surface. In 2015, the AI behind Google’s image search algorithm exhibited glaring racial bias. In 2023, ChatGPT creator OpenAI found itself in at least two severe legal battles: one with 17 prominent authors for copyright infringement and the other for violating provisions in the GDPR.
The problem seems new, and the stakes have gone higher. But as with all things with an ethical dimension, the solution will always boil down to the basics: transparency, best practices, good governance, risk management, and compliance.
Ethical Concerns Associated with AI and Machine Learning
Machine learning and artificial intelligence are digital technologies that enable machines to learn from large volumes of data and perform tasks that typically require human intelligence. Today, those tasks include writing computer code, giving legal advice, making music, generating digital art, and discovering new medical drugs. While extremely useful, these technologies raise ethical concerns:
- Bias: AI and ML systems are designed and deployed by humans who are not immune from having biases of their own. The technologies are also trained on data that may be biased as well. That can lead to unfair or discriminatory outcomes.
- Privacy: ML and AI systems may sometimes collect and process significant volumes of personal data. That raises concerns about privacy and data security.
- Safety: AI and machine learning systems are increasingly being used for high-risk applications such as transportation and medical surgery. There have been cases where such systems have malfunctioned and caused human deaths.
- Transparency: For many valid reasons, corporations who create AI systems typically restrict visibility into their core algorithms. Unfortunately, this lack of transparency can make it difficult to hold the systems accountable for costly and unwanted outcomes.
- Automated Decision Making: Many common services and functions (such as credit scoring, workforce recruitment, and healthcare) use ML and AI to automate processes that can significantly impact people’s lives. This practice can become problematic when the criteria and methodology for making decisions are not transparent, and accountability for negative outcomes needs to be clarified.
Governance and Ethics in Data Science and AI
Governance and ethics help ensure that data science and AI are used legally, responsibly, and ethically. They promote accountability and transparency, improve stakeholder engagement, mitigate risks, and drive compliance with relevant laws and standards.
Key data science and AI governance issues include collecting and handling sensitive data, discrimination, and bias in developing and using AI and ML systems, and how to prevent flawed AI-driven outcomes that negatively impact people in areas such as job opportunities, credit scoring, and health diagnosis.
Ethical AI and Machine Learning Resources
To address these concerns, it is crucial for stakeholders always to uphold ethical standards and adopt responsible, trust-based practices. Some of the existing regulations and guidelines for responsible AI and machine learning usage include:
- The General Data Protection Regulation (GDPR). This legislation protects the privacy and data rights of individuals. It requires transparency and consent for automated decision-making.
- The OECD Principles on Artificial Intelligence. These standards are agreed upon by participating governments to promote responsible stewardship of artificial intelligence that respects human rights and democratic values.
- The IEEE Ethically Aligned Design (EAD). This framework establishes high-level ethical principles for autonomous and intelligent systems.
AWS Resources for Responsible AI and Machine Learning
TrustNet partners with organizations like Amazon Web Services (AWS) that share our values and standards for the ethical use of data, AI, and machine learning. AWS provides resources that help companies integrate ethical practices in AI and machine learning.
Building Ethical AI
AI systems have been known to go haywire and lead to unwanted consequences. Hence, developing and training AI within a framework that upholds ethical values and principles such as transparency, fairness, accountability, privacy, and human rights is critical. Doing this can help:
- Enhance trust and confidence in AI systems among users, customers, and society.
- Reduce financial, legal, regulatory, and reputational risks associated with unethical or harmful AI systems.
- Improve social, environmental, and economic outcomes of AI systems.
Responsibly building AI entails a holistic approach that considers the ethical implications of AI at all stages of development, from design to deployment. These key steps can help achieve that:
- Engage all stakeholders. When conducting risk-benefit analysis or formulating policies, adopt a multidisciplinary approach that involves stakeholders from different fields such as business, law, technology, and ethics. Assign roles and responsibilities for designing and implementing the AI or ML system.
- Establish the values and principles (such as human rights and user privacy) that must be met in all AI or ML system aspects. Create a data and AI ethics checklist that includes data quality, data protection and privacy, fairness, transparency, and continuous monitoring. Set the red lines that must never be crossed.
- Embed ethical principles into the entire process, from design to deployment. Minimize bias by designing systems that use inclusive and diverse training data. Make transparency integral to system design and processes.
- Enforce the established standards.
- Continuously monitor, improve, and refine the ethical aspects of AI and ML systems.
Final Takeaway
The responsible and ethical use of data, machine learning, and AI helps ensure these technologies benefit society without causing severe side effects. The mechanisms behind AI and the issues and risks can be extremely complex.
But organizations can start with something simple: adopt best practices and implement proven methods that drive good governance.
Widely accepted frameworks such as NIST, GDPR, SOC 2, and ISO 27001 allocate a portion of their control requirements and objectives to ethics and good governance. That means complying with such security frameworks already covers significant ground regarding the ethical use of digital technologies — especially in data privacy and protection.
Additionally, consider adopting comprehensive solutions such as the iTrust Cyber Risk Ratings platform that provides 360-degree visibility into your security and compliance infrastructure. Partnering with a trusted GRC services provider like TrustNet can also unload much of the heavy lifting required for maintaining an ethical approach to the design, implementation, and usage of data, machine learning, and AI.
Significant technological advances have always been disruptive. However, successful businesses thrive amid disruption by proactively mitigating emerging risks while optimizing the game-changing benefits.